In a recent MIT Technology Review article, author Virginia Eubanks discusses her book Automating Inequality. In it, she argues that the poor are the testing ground for new technology that increases inequality— highlighting that when algorithms are used in the process of determining eligibility for/allocation of social services, it creates difficulty for people to get services, while forcing them to deal with an invasive process of personal data collection.
I’ve spoken a lot about the dangers associated with government use of face recognition in law enforcement, yet, this article opened my eyes to the unfair and potentially life threatening practice of refusing or reducing support services to citizens who may really need them— through determinations based on algorithmic data.
To some extent, we’re used to companies making arbitrary decisions about our lives – mortgages, credit card applications, car loans, etc. Yet, these decisions are based almost entirely on straight forward factors of determination— like credit score, employment, and income. In the case of algorithmic determination in social services, there is bias in the form of outright surveillance in combination with forced PII share imposed upon recipients.
Eubanks gives as an example the Pittsburg County Office of Children, Youth and Families using the Allegheny Family Screening Tool (AFST) to assess the risk of child abuse and neglect through statistical modeling. The use of the tool leads to disproportionate targeting of poor families because the data fed to the algorithms in the tool often comes from public schools, the local housing authority, unemployment services, juvenile probation services, and the county police, to name just a few— basically, the data of low income citizens who typically use these services/interact with them regularly. Conversely, data from private services such as private schools, nannies, and private mental health and drug treatment services — isn’t available.
Determination tools like AFST equate poverty with signs of risk of abuse, which is blatant classism— and a consequence of the dehumanization of data. Irresponsible use of AI in this capacity, like that of its use in law enforcement and government surveillance, has the real potential to ruin lives.
Taylor Owen, in his 2015 article titled “The Violence of Algorithms”, described a demonstration he witnessed by intelligence analytics software company Palantir, and made two major points in response— the first being that oftentimes these systems are written by humans, based on data tagged and entered by humans, and as a result are “chock full of human bias and errors.” He then suggests that these systems are increasingly being used for violence.
“What we are in the process of building is a vast real-time, 3-D representation of the world. A permanent record of us…but where does the meaning in all this data come from?” he asked, establishing an inherent issue in AI and datasets.
Historical data is useful only when it is given meaningful context, which many of these datasets are not given. When we are dealing with financial data like loans and credit cards, determinations, as I mentioned earlier— are based on numbers. While there are surely errors and mistakes made during these processes, being deemed unworthy of credit will likely not lead the police to their door.
However, a system built to predict deviancy, that uses arrest data as a main factor in determination, is not only likely to lead to police involvement — it is intended to do so.
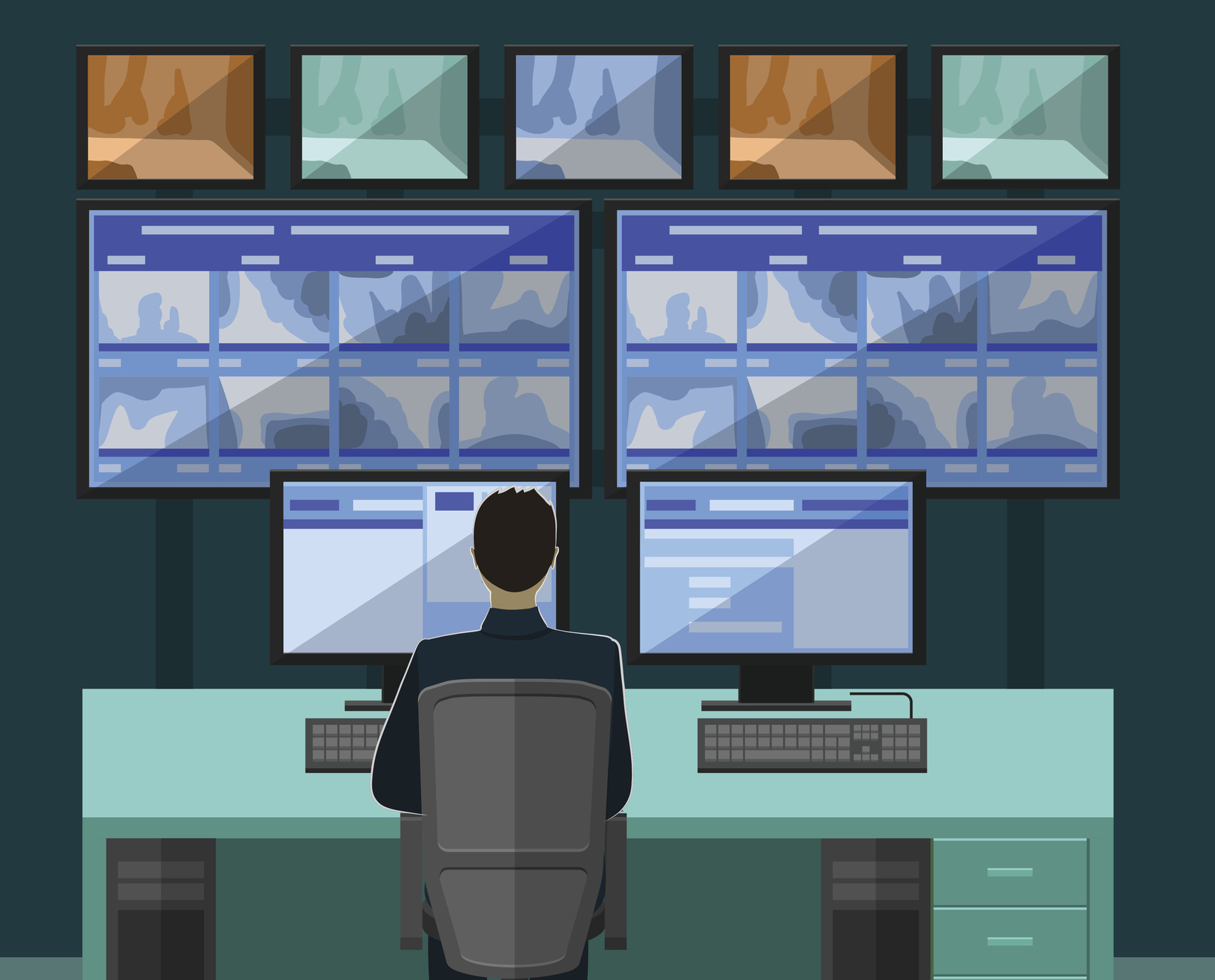
Image courtesy of Getty Images
When we recall modern historical policies which were perfectly legal in their intention to target minority groups, Jim Crow certainly comes to mind. And let’s also not forget that these laws were not declared unconstitutional until 1967, despite the Civil Rights Act of 1965.
In this context you can clearly see that according to the Constitution, Blacks have only been considered full Americans for 51 years. Current algorithmic biases, whether intentional or inherent, are creating a system whereby the poor and minorities are being further criminalized, and marginalized.
Clearly, there is the ethical issue around the responsibility we have as a society to do everything in our power to avoid helping governments get better at killing people, yet the lion’s share of this responsibility lies in the lap of those of us who are actually training the algorithms— and clearly, we should not be putting systems that are incapable of nuance and conscience in the position of informing authority.
In her work, Eubanks has suggested something close to a hippocratic oath for those of us working with algorithms— an intent to do no harm, to stave off bias, to make sure that systems did not become cold, hard oppressors.<
To this end, Joy Buolamwini of MIT, the founder and leader of the Algorithmic Justice League, has created a pledge to use facial analysis technology responsibly.
The pledge includes commitments like showing value for human life and dignity, which includes refusing to engage in the development of lethal autonomous weapons, and not equipping law enforcement with facial analysis products and services for unwarranted individual targeting.
This pledge is an important first step in the direction of self regulation, which I see as the beginning of a larger grass-roots regulatory process around the use of face recognition.
Source: Tech Crunch