Someday we’ll have an app that you can point at a weird bug or unfamiliar fern and have it spit out the genus and species. But right now computer vision systems just aren’t up to the task. To help things along, researchers have assembled hundreds of thousands of images taken by regular folks of critters in real life situations — and by studying these, our AI helpers may be able to get a handle on biodiversity.
Many computer vision algorithms have been trained on one of several large sets of images, which may have everything from people to household objects to fruits and vegetables in them. That’s great for learning a little about a lot of things, but what if you want to go deep on a specific subject or type of image? You need a special set of lots of that kind of image.
For some specialties, we have that already: FaceNet, for instance, is the standard set for learning how to recognize or replicate faces. But while computers may have trouble recognizing faces, we rarely do — while on the other hand, I can never remember the name of the birds that land on my feeder in the spring.
Fortunately, I’m not the only one with this problem, and for years the community of the iNaturalist app has been collecting pictures of common and uncommon animals for identification. And it turns out that these images are the perfect way to teach a system how to recognize plants and animals in the wild.

Could you tell the difference?
You might think that a computer could learn all it needs to from biology textbooks, field guides, and National Geographic. But when you or I take a picture of a sea lion, it looks a lot different from a professional shot: the background is different, the angle isn’t perfect, the focus is probably off, and there may even be other animals in the shot. Even a good computer vision algorithm might not see much in common between the two.
The photos taken through the iNaturalist app, however, are all of the amateur type — yet they have also been validated and identified by professionals who, far better than any computer, can recognize a species even when it’s occluded, poorly lit, or blurry.
The researchers, from Caltech, Google, Cornell, and iNaturalist itself, put together a limited subset of the more than 1.6 million images in the app’s databases, presented this week at CVPR in Salt Lake City. They decided that in order for the set to be robust, it should have lots of different angles and situations, so they searched for species that have had at least 20 different people spot them.
The resulting set of images (PDF) still has over 859,000 pictures of over 5,000 species. These they had people annotate by drawing boxes around the critter in the picture, so the computer would know what to pay attention to. A set of images was set aside for training the system, another set for testing it.
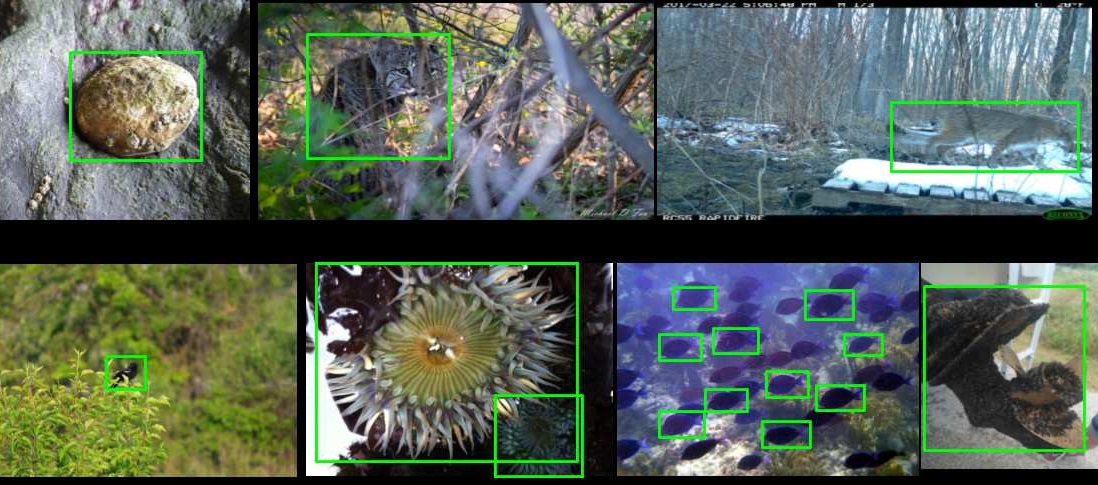
Examples of bounding boxes being put on images.
Ironically, they can tell it’s a good set because existing image recognition engines perform so poorly on it, not even reaching 70 percent first-guess accuracy. The very qualities that make the images themselves so amateurish and difficult to parse make them extremely valuable as raw data; these pictures haven’t been sanitized or set up to make it any easier for the algorithms to sort through.
Even the systems created by the researchers with the iNat2017 set didn’t fare so well. But that’s okay — finding where there’s room to improve is part of defining the problem space.
The set is expanding, as others like it do, and the researchers note that the number of species with 20 independent observations has more than doubled since they started working on the dataset. That means iNat2018, already under development, will be much larger and will likely lead to more robust recognition systems.
The team says they’re working on adding more attributes to the set so that a system will be able to report not just species, but sex, life stage, habitat notes, and other metadata. And if it fails to nail down the species, it could in the future at least make a guess at the genus or whatever taxonomic rank it’s confident about — e.g. it may not be able to tell if it’s anthopleura elegantissima or anthopleura xanthogrammica, but it’s definitely an anemone.
This is just one of many parallel efforts to improve the state of computer vision in natural environments; you can learn more about the ongoing collection and competition that leads to the iNat datasets here, and other more class-specific challenges are listed here.
Source: Tech Crunch